One-2-3-45:One Image to 3D Mesh in 45s
Abstract
Single image 3D reconstruction is an important but challenging task that requires extensive knowledge of our natural world. Many existing methods solve this problem by optimizing a neural radiance field under the guidance of 2D diffusion models but suffer from lengthy optimization time, 3D inconsistency results, and poor geometry. In this work, we propose a novel method that takes a single image of any object as input and generates a full 360-degree 3D textured mesh in a single feed-forward pass. Given a single image, we first use a view-conditioned 2D diffusion model, Zero123, to generate multi-view images for the input view, and then aim to lift them up to 3D space. Since traditional reconstruction methods struggle with inconsistent multi-view predictions, we build our 3D reconstruction module upon an SDF-based generalizable neural surface reconstruction method and propose several critical training strategies to enable the reconstruction of 360-degree meshes. Without costly optimizations, our method reconstructs 3D shapes in significantly less time than existing methods. Moreover, our method favors better geometry, generates more 3D consistent results, and adheres more closely to the input image. We evaluate our approach on both synthetic data and in-the-wild images and demonstrate its superiority in terms of both mesh quality and runtime. In addition, our approach can seamlessly support the text-to-3D task by integrating with off-the-shelf text-to-image diffusion models.
Overview
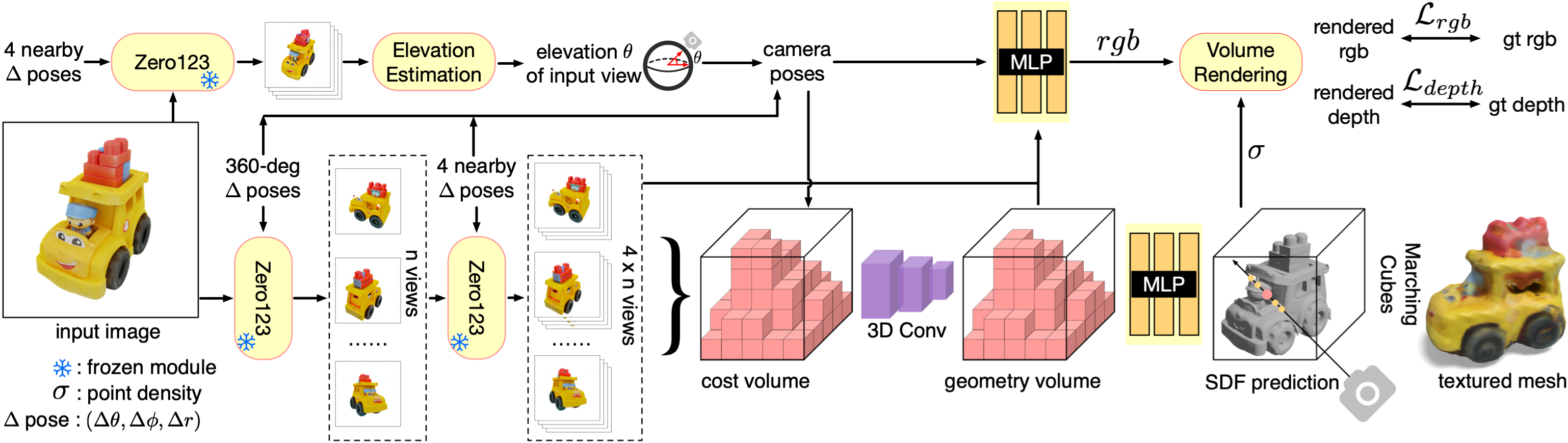
Our method consists of three primary components: (a) Multi-view synthesis: we use a view-conditioned 2D diffusion model, Zero123, to generate multi-view images in a two-stage manner. The input of Zero123 includes a single image and a relative camera transformation, which is parameterized by the relative spherical coordinates $(\Delta \theta, \Delta \phi, \Delta r)$. (b) Pose estimation: we estimate the elevation angle θ of the input image based on four nearby views generated by Zero123. We then obtain the poses of the multi-view images by combining the specified relative poses with the estimated pose of the input view. (c) 3D reconstruction: We feed the multi-view posed images to an SDF-based generalizable neural surface reconstruction module for 360◦ mesh reconstruction.
Problems of Zero123 Based Methods
Zero123 tends to generate predictions that are perceptually similar to the ground truth and have similar contours or boundaries, but the pixel-level appearance may not be exactly the same. Nevertheless, such inconsistencies between the source views are already fatal to traditional optimization-based methods. Thus, both traditional NeRF-based andor SDF-based methods failed to reconstruct high-quality 3D meshes from predictions of Zero123.
2-Stage Source View Selection and Groundtruth-Prediction Mixed Training
To enable the module to learn to handle the inconsistent predictions from Zero123 and reconstruct a consistent 360◦ mesh, both the ground-truth RGB and depth values are supervised during training.
Camera Pose Estimation
Zero123 predicts four nearby views of the input image at first. Then for each elevation candidate angle,
compute the corresponding camera poses for the four images and calculate a reprojection error for this set of camera poses to measure the consistency between the images and the camera poses in a coarse-to-fine manner. The elevation angle with the smallest reprojection error is used to generate the camera poses for all 4 × n source views by combining the pose of the input view and the relative poses.
Re-projection Error.
For each triplet of images $(a, b, c)$ sharing a set of keypoints $P$, we consider each point $p \in P$. Utilizing images $a$ and $b$, we perform triangulation to determine the 3D location of $p$. We then project the 3D point onto the third image $c$ and calculate the reprojection error, which is defined as the $l1$ distance between the reprojected 2D pixel and the estimated keypoint in image c. By enumerating all image triplets and their corresponding shared keypoints, we obtain the mean projection error for each elevation angle candidate